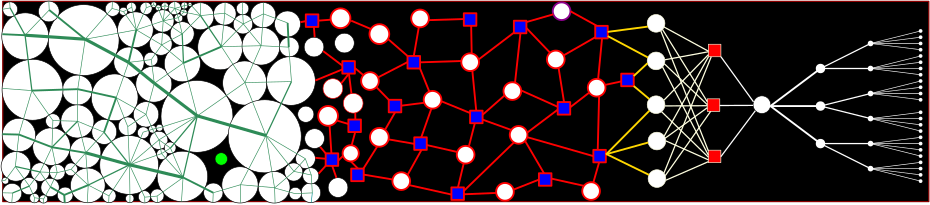
CNRS Researcher in theoretical physics
Université Paris-Saclay, CNRS, CEA,
Institut de Physique Théorique, Gif-Sur-Yvette, France.
I am a statistical physicist working on complex systems. In the past 10 years I focused on two main research lines: the physics of amorphous solids and high dimensional optimization problems, with application to machine learning and neuroscience.
Structural Glasses. I constructed of a theory of the glass phase starting from the soluble yet non-perturbative limit of infinite spatial dimensions. This has allowed: (i) the identification of a new phase transition within the glass phase, the Gardner transition; (ii) the exact characterization of the jamming transition; (iii) the development of a theory of amorphous solids under load and the corresponding yielding transition; (iv) the study of low temperature excitations.
Optimization, Continuous Constraint Satisfaction Problems, High-dimensional Inference and Machine Learning. I developed a research line to study continuous constraint satisfaction problems (CCSP), namely problems where a large number of constraints are imposed on a large set of continuous variables. I attacked these problems both studying the corresponding phase diagrams and, more extensively, studying practical search algorithms such as gradient descent and variants. Among the results that I obtained: (i) the study of search algorithms via dynamical mean field theory, in particular stochastic gradient descent; (ii) thedefinition of new classes of CCSP; (iii) the introduction of canyon landscapes and (iv) the solution of the trainining dynamics of two-layer neural networks.
Theoretical neuroscience. Biological neural networks are substantially different from their artificial counterpart. They are dynamical systems that are not described as optimizing any cost function. The resulting dynamics typically displays chaotic attractors. I recently developed a dynamical mean field theory to study how learning takes place in such settings.
Solution of structural glass models in infinite dimension:
- Gardner transition in structural glasses: mean field theory, RG analysis and experiments.
- Theory of the Jamming transition: computation of the critical exponents and characterization of marginal stability; jamming of non-spherical particles, upper critical dimension and hyperuniformity. Jamming critical phase in linear soft spheres. Avalanches. Rigidity transition in confluent tissues.
- Theory of the low temperature harmonic excitations of amorphous solids. Euclidean Random Matrices, Boson Peak and localized soft excitations.
- Theory of two level system excitations.
- Microscopic theory of rheology of amorphous solids: yielding as a critical spinodal with emerging RFIM criticality, shear-jamming of stable hard sphere glasses, two-step yielding, stability map of glasses, breakdown of elasticity at low temperatures.
- Dynamical Criticality: computation of the dynamical critical exponents at the MCT transition as well as at the Gardner point.
- Quasi-equilibrium approach to the dynamics of structural glasses.
Constraint satisfaction and optimization problems
- Jamming in multilayer neural networks.
- Emergence of isostatic marginally stable critical phases both in infinite and finite dimensional optimization problems. Theory of the corresponding non-linear excitations.
- Dynamical mean field theory for gradient-based optimization.
High-dimensional statistical inference and machine learning
- Glassy nature of the hard phase of inference problems.
- Emergence of a generic gap between gradient based algorithms and message passing ones.
- Analysis of gradient descent dynamics in high-dimensional inference problems. Momentum-based minimization algorithms.
- Dynamical mean field theory for stochastic gradient descent. Persistent-SGD. Effective temperature.
- Replica-symmetry-breaking implementation of Approximate Message Passing algorithms.
- Introduction of canyon landscapes.
- Solution of the dynamics of large overparametrized two-layer neural networks. See the recent talk.
Learning in Recurrent Neural Networks
- Tuning chaos through Hebbian plasticity.
- DMFT analysis of FORCE algorithm.
- Generative modelling via chaotic dynamics
Disordered high dimensional optimal control
- Analysis of controlled many-body dynamical systems
- Dynamical mean field theory of many-body HJB equations.
Field theory and renormalization
- Field theory for the glass transition. Dynamical heterogeneities at the glass transition.
- Non-perturbative renormalization group approach to instantons.
- Field theory for soft anharmonic spin glasses in a field.
- Scenario for the spin glass transition in a field: appearance of finite density of non-linear excitations.
G. Parisi, P. Urbani, F. Zamponi. Theory of simple glasses. Cambridge University Press 2020.
HDR manuscript, 2024, Slides
IPhT Lectures on disordered and glassy systems (video)
Les Houches Lectures on dynamics in high dimension (2020) (lecture 1, lecture 2, lecture 3)
Lectures at the School on Disordered Elastic Systems, São Paulo, Brazil, 2022 (Lecture 1, Lecture 2, Lecture 3, Lecture 4)
IPhT Lectures: Generative models in a nutshell, postponed.
- Statistical Physics and Condensed Matter seminars of the IPhT
- IPhT Theoretical Physics courses
- Machine Learning and Statistical Physics @ Plateau
- Invited talk at the Simons Institute for the theory of computing, 2025
- Invited talk at the ASCAI meeting, Orsay, Institut de Mathematique, 2025
- Invited talk at the Lausanne event on Machine Learning and neural network theory, 2025
- Invited talk at workshop “Learning and Optimization in High Dimensions”, 2025
- Invited talk the workshop “Roc(k)in’AI 2.0”, Roccella Ionica, Italy, 2025